Panasonic Made a New Super Accurate In-Camera Subject Recognition AI
Panasonic announced that it developed a new image recognition AI that has a new “classification algorithm” that is more accurate than conventional methods.
While subject recognition technology from most camera manufacturers has become quite good, there is still room for improvement. For example, Panasonic notes that while AI is able to classify subjects into categories based on their appearance, it can’t do a very good job of being specific within those categories.
While a camera can see a “train” or a “dog,” for example, it doesn’t necessarily have the ability to sub-categorize those findings into a specific breed or model of train. It also might not be able to tell the difference between subjects that look similar to one another but are actually different.
“Furthermore, there are many cases in which the same object can appear to look different due to differences in shooting conditions such as orientation, weather, lighting, or background,” Panasonic says.
“It is important to consider how best to deal with such diversity in appearance. In order to improve the accuracy of image recognition, research to this point has been carried out with the aim of achieving robust image recognition that is not affected by diversity, and classification algorithms have been devised to find similarities within subcategories and features common to objects in a given category.”
The company says that with conventional methods, the ability for current object recognition tools has reached a limit to what they can do and AI often has trouble successfully recognizing objects and subjects as being in the same category, which Panasonic says results in a decrease in recognition accuracy.
To that end, it has developed a new technology that takes advantage of the differences in appearance, resulting in a new training program called REAL-AI.
With current deep-learning frameworks, AI models essentially learn that things that look similar belong in the same categories. While helpful, it isn’t necessarily accurate. With the advancement of AI, though, it has now become possible to significantly increase the number of variations that can be taught during the training process.
As a result, it is now possible to teach an AI that certain objects or subjects belong in the same category even if they have significantly different appearances due to the subject itself (think how different breeds of dog can look) or if appearances are altered due to a camera’s orientation, the lighting on a scene, or the background.
“The distribution of appearances within a category is actually not uniform. Within the same category, there are multiple subcategories with multiple different trends in appearance (multimodal distribution). For example, in the ‘Birds’ category shown in Figure 1 (below), there are groups of images of the same bird with different tendencies, such as ‘birds flying in the sky’, ‘birds in the grassland’, ‘birds perched in trees’, and ‘bird heads’,” Panasonic explains.
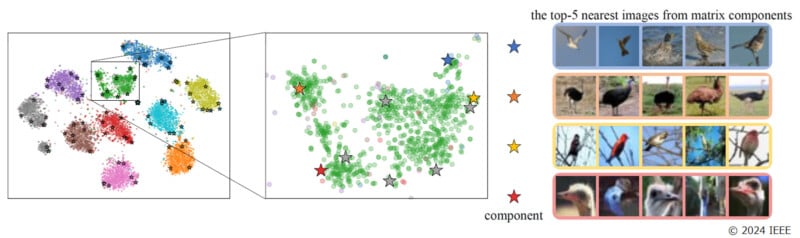
“Each of these images contains rich information about the object. If we focus on the essential features, we end up throwing away the diverse information that the images contain. Therefore, we have developed an algorithm that actively utilizes information about the various ways in which objects appear to improve AI’s ability to recognize images with multimodal distribution, which is difficult for AI. In order to continuously capture the distribution of features, we expanded the weight vector of the classification model, which has traditionally only been a one-dimensional vector, to a two-dimensional orthonormal matrix. This allows each element of the weight matrix to represent a variation of the image (differing background colors, object orientation, etc.).”
Panasonic further explains in the figure below that current AI has a difficult time understanding the difference between a “bus” and a “streetcar” due to their similar appearance. However, using its new algorithm, the company says that the AI was able to tell the difference and sub-categorize them (seen below in Figure 2).
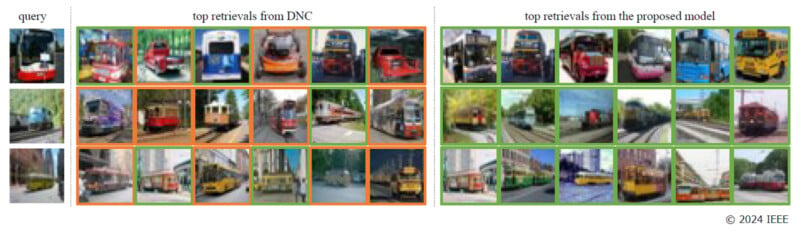
Despite this increase in reliability and accuracy, Panasonic says that its new algorithm only increases memory usage needs by 0.1% in practical use cases, meaning integration into cameras would require minimal hardware improvement.
Panasonic will continue to develop this new AI for practical application in the future. The full research paper on the development is available on a publicly accessible web page.
Image credits: Panasonic Holdings Co., Ltd., IEEE