Researchers Have Created an AI-Powered Mind Reader
Researchers from The University of Texas at Austin have published a study that describes a brain-computer interface that can decode continuous language from non-invasive brain recordings. In other words, an interface that can decode someone’s thoughts into a word sequence.
As reported by World Journal Post, the study describes a decoder that “generates intelligible word sequences that recover the meaning of perceived speech, imagined speech, and even silent videos, demonstrating that a single decoder can be applied to a range of tasks.”
The research team, comprising Jerry Tang, Amanda LeBel, Shailee Jain, and Alexander G. Huth, adds that their brain-computer interface “should respect mental privacy” and requires subject cooperation to achieve successful decoding, both in terms of training the decoder and applying it to a subject.
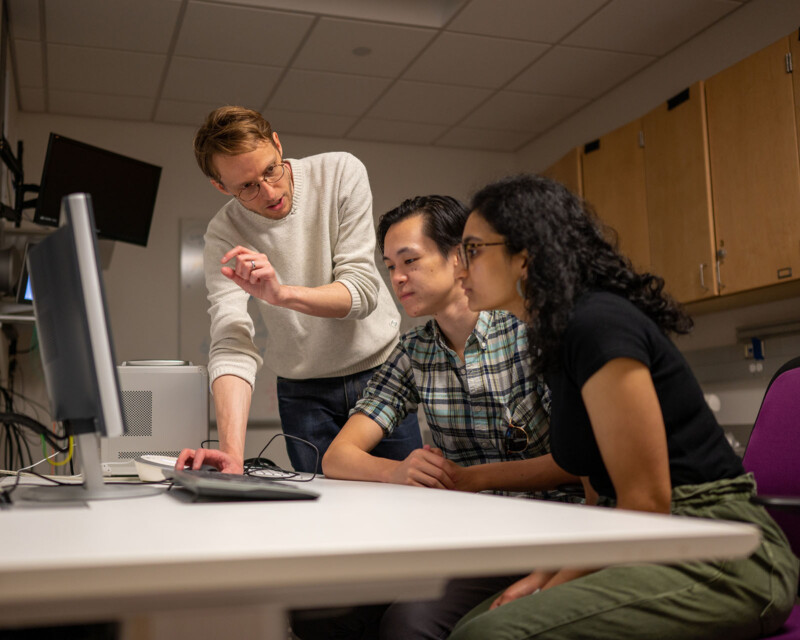
How the Research Differs From Prior Studies
While the concept of an AI-powered system that can read a person’s mind may seem farfetched — and perhaps a bit disturbing — similar research has used neural networks to help restore communication skills to people who have lost the ability to move or speak. In prior instances, AI has been used to interpret attempted communication, including replicating handwriting in a subject who can perform only twitches or micromotion. By imagining the act of writing, AI-assisted technology has successfully decoded intentionality to generate handwriting for a subject who cannot physically move.
What separates the new work from other language decoding systems is that it’s non-invasive — the system doesn’t require any surgical implants. Participants also don’t need to think about words from any prescribed list, a limitation of some other language decoding systems.

In the new study by Tang, LeBel, Jain, and Huth, participants were asked to imagine speech, and using fMRI scans, the technology delivered reasonably accurate recreations. Furthermore, and perhaps more impressively, subjects were shown a silent film and asked to think about it. The AI-powered interface exhibited the ability to turn thoughts into semantic language.
“This isn’t just a language stimulus. We’re getting at meaning, something about the idea of what’s happening. And the fact that that’s possible is very exciting,” explains Huth, a neuroscientist at the University of Texas.
“For a noninvasive method, this is a real leap forward compared to what’s been done before, which is typically single words or short sentences. We’re getting the model to decode continuous language for extended periods of time with complicated ideas,” continues Huth.
Decoding System Shows Promise in Limited Study
While exciting, the research remains limited at this point. The study involved just three participants, who were used to help train a language model according to fMRI scans. Each participant was in Dr. Huth’s lab for 16 hours and primarily listened to several narrative podcasts. As the participants listened, the scanner recorded changing blood oxygenation levels in different parts of their brains. The recorded patterns were then matched to the words and phrases that each participant heard.
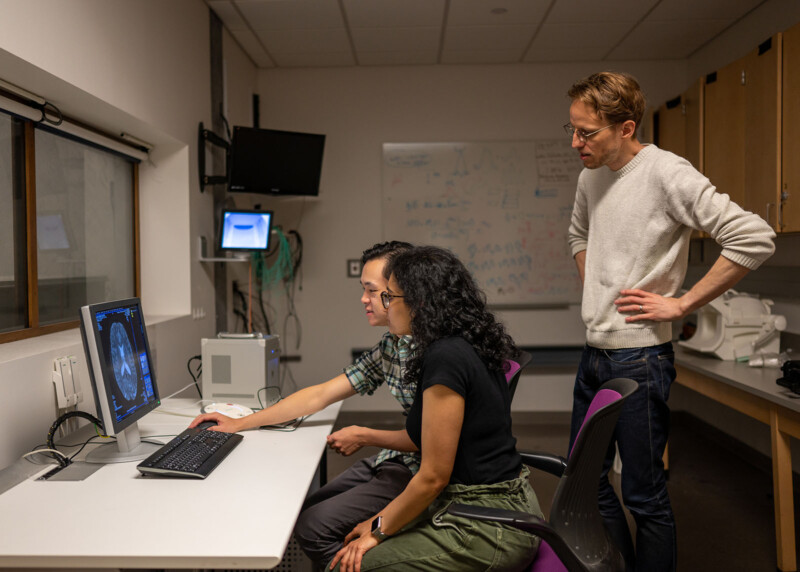
As part of their study, Huth and the others then used an AI model to translate participants’ fMRI images into semantics. The decoding system was subsequently tested by having participants listen to new recordings and comparing the decoded translation against the actual transcript of the audio.
While the exact wording generated by the decoder doesn’t match the transcript, the system preserved meaning with impressive precision. About “half the time,” the trained decoder closely matched the intended meaning.
For example, the original transcript writes, “I got up from the air mattress and pressed my face against the glass of the bedroom window expecting to see eyes staring back at me but instead only finding darkness.”
The decoded language built upon measured brain activity is, “I just continued to walk up to the window and open the glass I stood on my toes and peered out I didn’t see anything and looked up again I saw nothing.”
Another test asked participants to imagine telling a story inside their minds. Afterward, the participant recited the story for reference.
“Look for a message from my wife saying that she had changed her mind and that she was coming back,” a participant imagined.
The decoder produced the following text, “To see her for some reason I thought she would come to me and say she misses me.”
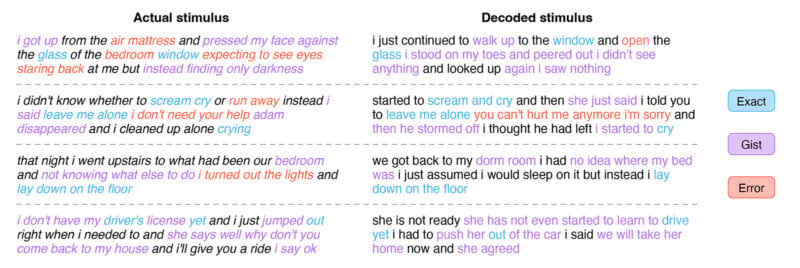
Returning to the part of the study when participants watched a silent movie, the language-decoding method delivered a rough synopsis of the silent film people watched based on the data from an fMRI scan being decoded and turned into language.
Greta Tuckute, a neuroscientist at the Massachusetts Institute of Technology, who was not involved in the study, remarks that the new research shows promise and suggests that perhaps in some ways, meaning can be decoded from brain activity.
Another neuroscientist not involved in the study, Shinji Nishimoto of Osaka University, adds that “brain activity is a kind of encrypted signal, and language models provide ways to decipher it.” Nishimoto also remarks that the study’s authors show that “the brain uses common representations across” multiple processes, including externally driven language processes and internal imaginative action.
However, the “common representations” that Nishimoto mentions are not necessarily common among all people, but rather, common to an individual.
Practical and Ethical Concerns, and How the Research Could Help People
The authors highlight some limitations of their work, including that model training is tedious and must be performed on an individual. One person’s map of brain activity to semantic meaning is not believed to be easily transferrable to someone else. For example, how one person’s brain encodes words and thoughts does not necessarily reflect anyone else’s brain activity. The researchers tried to use a decoder trained on one participant to read someone else’s activity, and it failed. It appears that each person uniquely represents meaning.
Further, fMRI scanners are bulky and expensive. It is not something a person could wear while going about their daily life, at least not yet.
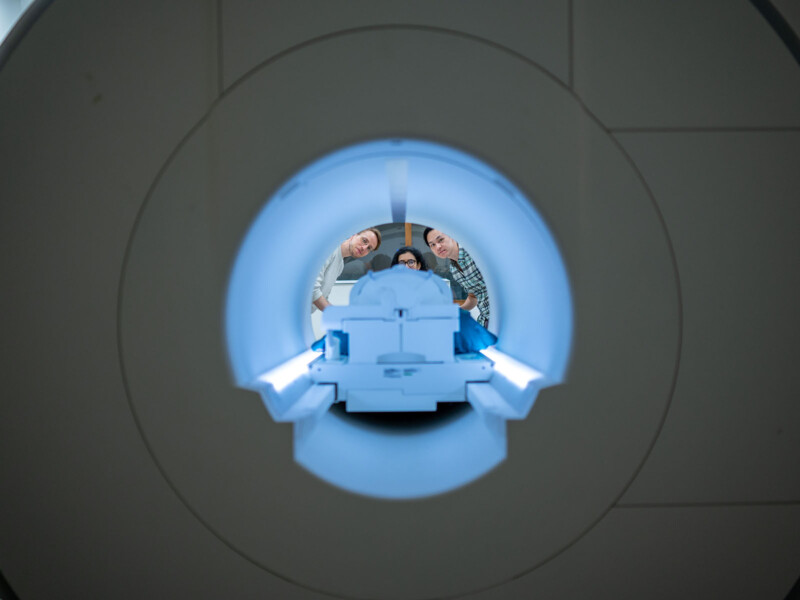
Another limitation should offer solace to those worried about malicious mind-reading devices. Participants must be willing to have their brain activity accurately measured and deciphered. At least for now, the machine can’t overcome someone sabotaging the process by thinking about something else. The decoder could not decode everything someone thought.
“We take very seriously the concerns that it could be used for bad purposes and have worked to avoid that,” Tang says. “We want to make sure people only use these types of technologies when they want to and that it helps them.”
Despite these limitations, the study shows immense promise. Technology may advance and be practical enough to help those who cannot speak or write communicate more effectively.
Image credits: Header photo licensed via Depositphotos.