Google’s New AI Photo Upscaling Tech is Jaw-Dropping
Photo enhancing in movies and TV shows is often ridiculed for being unbelievable, but research in real photo enhancing is actually creeping more and more into the realm of science fiction. Just take a look at Google’s latest AI photo upscaling tech.
In image super-resolution, a machine learning model is trained to turn a low-res photo into a detailed high-res photo, and potential applications of this range from restoring old family photos to improving medical imaging.
Google has been exploring a concept called “diffusion models,” which was first proposed in 2015 but which has, up until recently, taken a backseat to a family of deep learning methods called “deep generative models.” The company has found that its results with this new approach beat out existing technologies when humans are asked to judge.
The first approach is called SR3, or Super-Resolution via Repeated Refinement. Here’s the technical explanation:
“SR3 is a super-resolution diffusion model that takes as input a low-resolution image, and builds a corresponding high resolution image from pure noise,” Google writes. “The model is trained on an image corruption process in which noise is progressively added to a high-resolution image until only pure noise remains.
“It then learns to reverse this process, beginning from pure noise and progressively removing noise to reach a target distribution through the guidance of the input low-resolution image.”
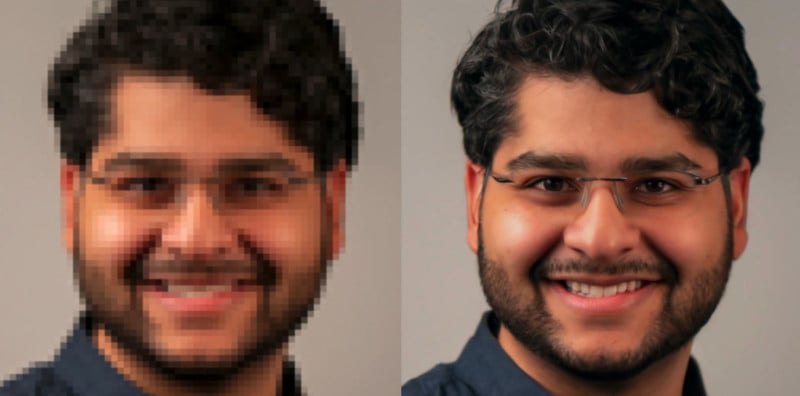
SR3 has been found to work well on upscaling portraits and natural images. When used to do 8x upscaling on faces, it has a “confusion rate” of nearly 50% while existing methods only go up to 34%, suggesting that the results are indeed photo-realistic.
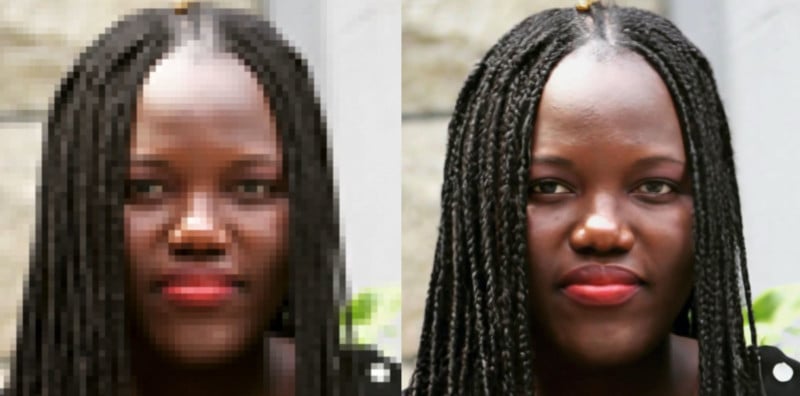
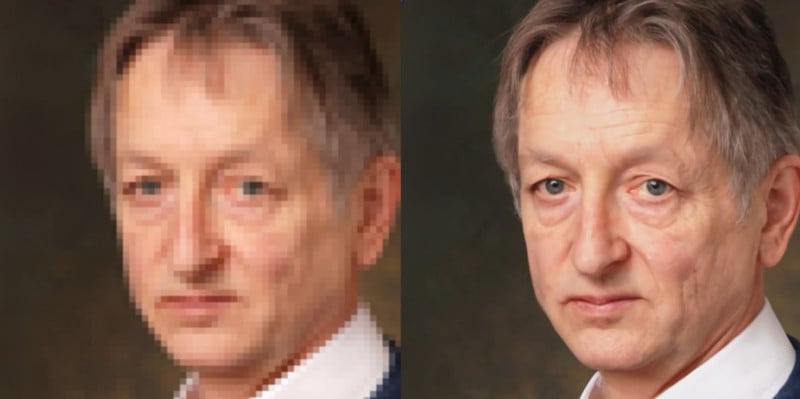
Here are other portraits upscaled from low-resolution originals:
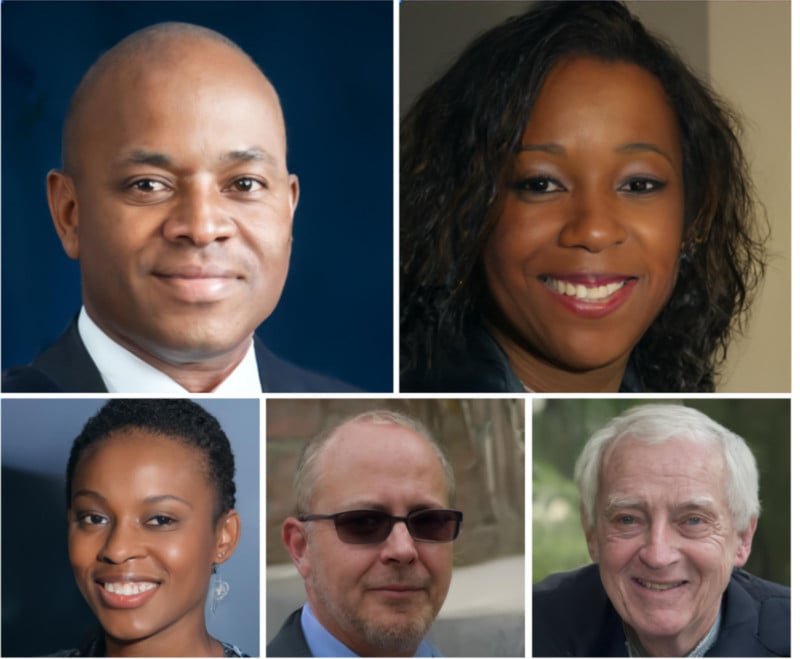
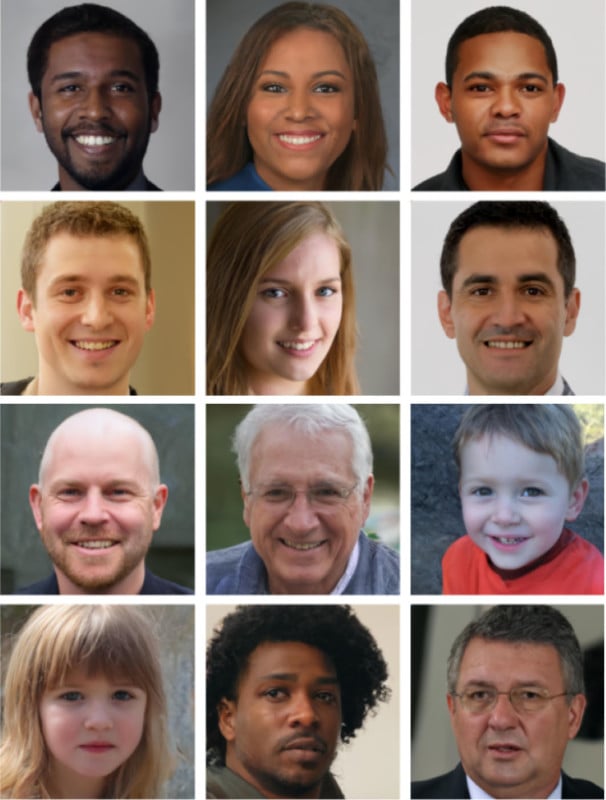
Once Google saw how effective SR3 was in upscaling photos, the company went a step further with a second approach called CDM, a class-conditional diffusion model.
“CDM is a class-conditional diffusion model trained on ImageNet data to generate high-resolution natural images,” Google writes. “Since ImageNet is a difficult, high-entropy dataset, we built CDM as a cascade of multiple diffusion models. This cascade approach involves chaining together multiple generative models over several spatial resolutions: one diffusion model that generates data at a low resolution, followed by a sequence of SR3 super-resolution diffusion models that gradually increase the resolution of the generated image to the highest resolution.”
Google has published a set of examples showing low-resolution photos upscaled in a cascade. A 32×32 photo can be enhanced to 64×64 and then 256×256. A 64×64 photo can be upscaled to 256×256 and then 1024×1024.
As you can see, the results are impressive and the final photos, despite having some errors (such as gaps in the frames of glasses), would likely pass as actual original photographs for most viewers at first glance.
“With SR3 and CDM, we have pushed the performance of diffusion models to state-of-the-art on super-resolution and class-conditional ImageNet generation benchmarks,” Google researchers write. “We are excited to further test the limits of diffusion models for a wide variety of generative modeling problems.”