Scientists Say Deepfakes Can Take on Human Heartbeats Making Them Impossible to Detect
Generative AI technology has undermined what is real and what is not. The old remark That’s Photoshop — previously used to question an image’s authenticity — has now been replaced by That’s AI.
And as the tech gets better and better, it is harder to know whether something is AI-generated. Worse still, there is no real way of determining for certain whether a piece of content is synthetic, people who are well-versed in media can tell but there are fewer smoking guns.
Take deepfakes, for example, a previously reliable sign of authenticity is whether a video shows physiological signals such as a visible pulse. But a new study from Humboldt University of Berlin says that even that can now be faked.
The research published in Frontiers in Imaging found that some modern deepfake models can produce videos that show human-like heart rate indicators. Detection tools that rely on identifying these subtle signals, such as a pulse, misclassified fake videos as genuine.
“Here we show for the first time that recent high-quality deepfake videos can feature a realistic heartbeat and minute changes in the color of the face, which makes them much harder to detect,” says Peter Eisert, a professor at Humboldt and the study’s lead author, per Popular Science.
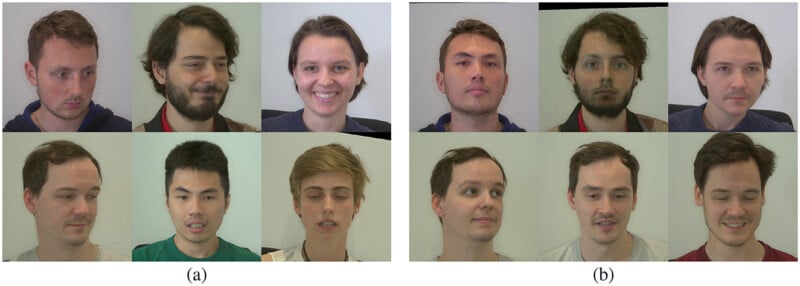
The study highlights a new challenge in the ongoing struggle to detect synthetic media. Deepfakes use AI to create manipulated images, videos, or audio files that can appear convincingly real. While some uses are benign, the technology has drawn criticism for enabling the spread of non-consensual explicit material. According to a 2023 report in Wired, more than 244,000 deepfake porn videos were uploaded to the top 35 such websites in a single week. Tools that make it easy to insert someone’s face into explicit content have made the issue more widespread.
Deepfakes have also raised concerns around misinformation and fraud. Fabricated videos of public figures, both famous and not-so-famous, have circulated widely. To address the growing problem, the U.S. Congress recently passed the Take It Down Act, which criminalizes the sharing of nonconsensual sexual imagery, including those generated by AI.
Efforts to detect deepfakes have traditionally relied on spotting visual inconsistencies like unnatural blinking or warped facial features. More recent systems have used remote photoplethysmography (rPPG), a method originally developed for telehealth, to detect signs of a heartbeat by analyzing light changes in facial skin.
To test whether this method still works, Humboldt researchers trained a detection model using real videos of participants performing various tasks. After analyzing just 10 seconds of footage, the system could reliably identify each person’s heart rate. However, when the same method was applied to deepfake versions of those participants, the results were surprising: the detector identified heartbeats in the manipulated videos and marked them as authentic.
“Our experiments demonstrated that deepfakes can exhibit realistic heart rates, contradicting previous findings,” the researchers say.
The deepfakes in the study weren’t deliberately programmed to simulate a heartbeat. Instead, the researchers believe the synthetic clips unintentionally “inherited” pulse-like signals from the original footage. Visual data from both the real and fake videos showed nearly identical light transmission patterns, suggesting these subtle signals were transferred during the video generation process.
“Small variations in skin tone of the real person get transferred to the deepfake together with facial motion, so that the original pulse is replicated in the fake video,” Eisert explains.
Although the study points to a gap in current detection systems, the researchers say the situation isn’t hopeless. Today’s deepfakes still fall short of replicating more complex patterns in blood flow across a person’s face over time. Other detection methods — such as tracking changes in pixel brightness or using digital watermarks — are being explored by tech companies like Adobe and Google to supplement traditional approaches.
Still, the findings highlight the need for continuous updates to detection technology. As Eisert and his team suggest, no single indicator may be sufficient on its own for long.
Image credits: Header photo licensed via Depositphotos.